IBM’s Impact On Fantasy Sports Gives Insight Into Future Of Consumerism
Add bookmark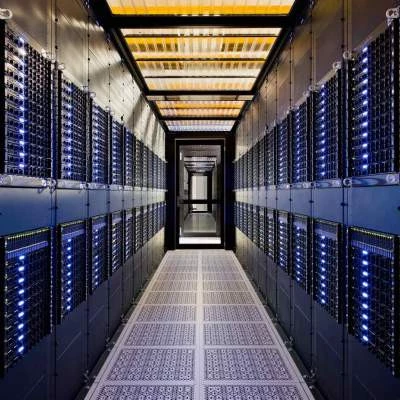
For the last three years, IBM Watson, the cognitive computing platform that can answer natural language questions (and international Jeopardy champion), has been providing ESPN with fantasy football predictions.
Millions of ESPN consumers (whether they know it or not) relied on IBM Watson during the 2019 NFL regular season, counting on Watson’s AI for accurate intel on which players to select in their starting lineup. But they didn’t just rely on statistical analysis from Watson.
IBM had improved its natural language processing algorithms to include more unstructured data and conversations that Watson pulled from the web.
ESPN's Daniel Dopp, co-host of "The Fantasy Show with Matthew Berry" on ESPN+ recently sat down with IBM engineer, Aaron Baughman at the IBM Innovation Lab to discuss Watson's disruption in the esoteric science of fantasy football – but his ideas extend way further than fantasy football, or even sports for that matter.
At the event, Dopp reflected on ESPN’s eagerness to partner with IBM Watson because the fantasy football audience, estimated to be around 10 million consumers, was a sizable chunk of ESPN's core readership.
Baughman brought up an interesting point that perfectly depicts the correlation between artificial and human intelligence… they’re codependent.
(For example, I’ve never solely relied on Watson’s fantasy predictions to set my lineup. Like any self-declared expert, I took the projections with a heavy grain of salt. But then again, maybe that's why I fought tooth and nail to avoid last place).
“It helps to have a compromise between the heart [humans] and the brain [AI]”
"It helps to have a compromise between the heart [meaning humans] and the brain [AI]. We trained Watson on millions of fantasy football stories, blog posts and videos. We taught it to develop a scoring range for thousands of players with their upsides and their downsides. And we taught it to estimate the chances a player will exceed their upside or fall below the downside," Baughman said.
Watson uses a word-embedding-system, a type of word representation that allows words with similar meaning to have a similar representation through automated, natural language processing.
During Watson’s development, the technology was fed 10 football dictionaries as well as 5,568,714 documents worth of football information from thousands of different sources, including content people were talking about close to real time (i.e. websites, podcasts and videos) using NLP techniques to literally learn the game.
The way it works in fantasy football, as told by sportechie: if IBM plugged in Travis Kelce, for example, Watson should show that he plays for the Chiefs. If it plugged in “Travis Kelce is to the Chiefs as Alvin Kamara is to…” it would come back with the Saints.
Watson predictions extending beyond sports
Watson produces 1,000 simulations (or potential outcomes) for the top 400 fantasy football players and over two billion insights... each day.
It’s no coincidence that the IBM data scientist's fantasy football team ran the table, going undefeated in the regular season, putting up an effortless 13-0 record. "No exaggeration," says Baughman.
But unless you’re an avid fantasy football player, or sports gambling addict, for that matter, why should we care?
According to IBM:
“That same thing [the impact IBM Watson had on fantasy football] can often be said for lawyers and doctors and businesspeople, but ideally, what both IBM developers and Watson are learning through fantasy football could be applied to all of those fields and more. If Watson can be taught football, it can also be taught to read, say, patient histories, helping to correlate patterns that medical professionals might not see as clearly otherwise. It could also sort through thousands of pages of legal or financial documents the way it worked through thousands of football-related articles and social-media posts - the tax-preparer H&R Block, for instance, is already using Watson to help it sort through changes in the tax code and make recommendations to its clients.”
As I mentioned in a recent CCW article, IBM estimates that 90% of the world's online data has been created in the past two years.
In other words (with content being churned out at a rapidly exponential rate), google (as one general example) is becoming difficult to find accurate or actionable information used to help both businesses and customers make predictions.
The same concept applies to any abundance of knowledge that’s difficult to sort through variables and generate predictions out of: sports statistics, patient history, financial statements, legal documents, customer data, etc.
According to Noah Syken, vice president of sports and entertainment partnerships at IBM, businesses are “increasingly relying on the rows and columns and numbers of structured data, but they don't tap into, 'What are people saying about my business and my company?' If you really want to understand what's happening in your business, you have to be able to extract all that unstructured information," that natural language processing technologies like Watson can provide.
As Jeanne Bliss, CCW Digital advisory board member, bestselling author, and former CX leader at Microsoft told us in a recent CCW report, “Only then can all areas being addressed separately and in silos like AI, social data and even experience efforts come together in a manner that a customer can see, feel and appreciate…”
0-100 Accelerator
And she’s right.
IBM Advertising Accelerator, which launched last week at the 2020 Consumer Electronics Show, leverages AI to predict the element combinations that drive the highest audience engagement and conversion. Watson automatically creates campaigns for customer segments using predictive and search models, with an eye to helping advertisers better understand customer preferences.
Advertising Accelerator tries to sniff out the optimal mix of creative marketing content while at the same time discovering new audiences based on message resonance. It analyzes each campaign from a creative and audience standpoint, surfacing insights on the highest- and lowest-performing experiences. And it continuously learns from past projects and deployments to improve its future recommendations and avoid the sack.